Bayesian statistics
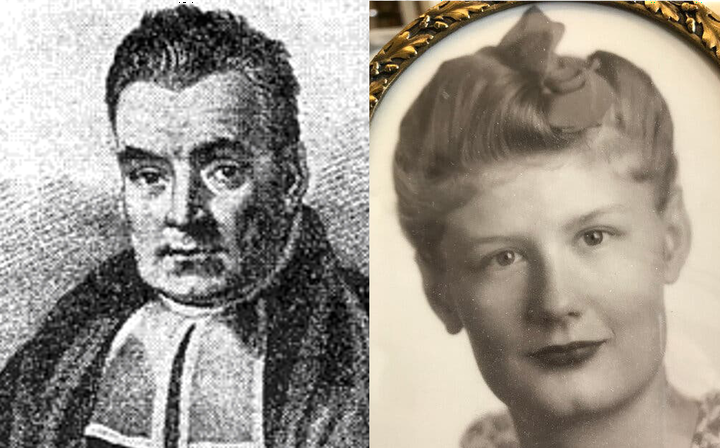
Statistical inference is at the core of scientific research because it allows us to learn in a systematic manner from data. Null hypothesis significance testing (NHST) is one way to learn from data, but it happens not to be my preferred way. This is not rooted in some deep philosophical insight, but in the observation that forcing research questions into the NHST framework is typically not that helpful for the typ of research I am doing.
In research on decision making theoretical progress is more readily achieved by the formulation of quantitative models. When such models are reasonably complex, Bayesian estimation more reliably estimates model parameters than alternative approaches. Just as importantly, Bayesian estimation as for example done in Stan also signals when model parameters cannot be estimated reliably.
In epidemiological research with very large data set effect sizes matter, because practically irrelvant effect sizes can reach statistical significance. Using observational data will often require complex models that do justice to the complexity of the data generating process. So even if Bayesian estimation of large epidemiological data sets can be much slower, I still prefer to wait for results I think I can trust more.
So far, I have published a few papers that uses Bayesian estimation. Click the “Bayesian” button below to see those.
If time permits, I also answer the one or the other question on the the Stan user forum.